Afsaneh Doryab
Assistant Professor, Systems Engineering
Assistant Professor, Computer Science
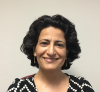
About
My research is at the intersection of ubiquitous computing, AI, HCI, and health. I work on computational modeling of human behavior (incl. Activity Recognition) from data streams collected via mobile, wearable, and embedded sensors.
Education
Faculty, School of Computer Science, Carnegie Mellon University, 2012-2019
Ph.D., Computer Science and Information Technology, Copenhagen, 2011
Research Interests
Ubiquitous and Mobile Computing
AI, Machine Learning, Data Mining
Intelligent Human-Centered Computing
Smart Health and Smart Cities
Selected Publications
Modeling Biobehavioral Rhythms with Passive Sensing in the Wild: A Case Study to Predict Readmission Risk after Pancreatic Surgery (Presented at the ACM Ubicomp conference, 2019)
Proceedings of the Acm on Interactive, Mobile, Wearable and Ubiquitous Technologies
ABS
Identifying Behavioral Phenotypes of Loneliness and Social Isolation with Passive Sensing: Statistical Analysis, Data Mining and Machine Learning of Smartphone and Fitbit Data, 2019
Jmir Mhealth and Uhealth
ABS
Estimation of symptom severity during chemotherapy from passively sensed data: exploratory study (Best paper Selected for the 2019 Edition of the IMIA Yearbook, Section Cancer Informatics)
Journal of Medical Internet Research
ABS
If It’s Convenient: Leveraging Context in Peer-to-Peer Variable Service Transaction Recommendations (Presented at the ACM Ubicomp conference, 2017)
In the Proceedings of the Acm on Interactive, Mobile, and Ubiquitous Technologies
ABS
Identifying Symptoms Using Technology. In: Moreno M., Radovic A. (eds) Technology and Adolescent Mental Health
Springer, Cham, 2018
ABS
Featured Grants & Projects
NSF-IIS-1816687: Computational Modeling of Human Rhythms to Improve Health and Quality of Life
According to recent research, balance is a significant contributor to health and well-being. Health challenges are associated with work and personal imbalances, in the form of overload, that exceed human capacity to manage their own natural pace. Part of these overloads is due to the imposition of activities and mistimed or incompatible demands on biological and psychological needs, or lack of attention to synchronization or receptivity of mental and physical states when meeting work and life demands. Advice about life management and health is often generic (for example, have 8 hours of sleep a day) rather than tailored to individual lifestyles. This research proposes to improve health and wellbeing in individuals through awareness of their personal rhythms, which are repeated cycles of internal and external events including biological, mental, social, and environmental. The investigators seek to design and evaluate a data analytic and modeling method to make people aware of what they could be doing at any given time to align with their biological clock and to achieve high performance in daily life and work without burning out or developing stress-related disorders. The research provides an intelligent platform for collecting longitudinal data and modeling human rhythms to enable detection of current, and prediction of future states in each rhythm to provide optimal action recommendations and interventions. The work leverages advances in wearable devices, sensing technology, and online sources to proactively collect and analyze physiological, psychological, behavioral, social, and environmental data to identify personal rhythms, to explore their relationship to positive physical, mental, and behavioral outcomes, and to provide people with tools to reason about these relationships and to improve outcomes. In addition to advancing the state of the art in modeling rhythmic patterns, the research will demonstrate the impact of rhythm-aware technology in changing people?s perceptions and behavior and directing them towards a more balanced and successful life. The integration of personal rhythms in the daily lives of individuals will transform societal activities and the overall performance of people and contributes to creating a healthier society.
Read More
Campus Life, Assessment of Student Health and Success
A cross-institution collaboration with multiple universities incl. Georgia Tech, Cornell, Dartmouth, Carnegie Mellon, and the University of Washington to assess students health and performance from passive mobile data. Led two semester-long data collection studies with 450 undergraduate students at CMU.
Context-aware Peer to Peer Exchange
An NSF funded project in collaboration with Penn State University and PARC to connect people in communities and help them exchange services and resources through a context-aware mobile infrastructure.
NeighborGenie App