Cong Shen
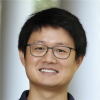
About
Cong Shen received his B.E. and M.E. degrees from the Department of Electronic Engineering, Tsinghua University, China. He received the Ph.D. degree from the Electrical Engineering Department, University of California Los Angeles (UCLA). He is currently an Assistant Professor of the Electrical and Computer Engineering Department at University of Virginia (UVa). Prior to joining UVa, He was a professor in the School of Information Science and Technology at University of Science and Technology of China (USTC). He also has extensive industry experience, having worked for Qualcomm Research, SpiderCloud Wireless, Silvus Technologies, and Xsense.ai, in various full time and consulting roles. His general research interests are in the area of machine learning and wireless communications. In particular, his current research focuses on multi-armed bandit, reinforcement learning, federated learning, and their engineering applications.
He received the NSF CAREER award in 2022. He was the recipient of the Best Paper Award in 2021 IEEE International Conference on Communications (ICC), and the Excellent Paper Award in the 9th International Conference on Ubiquitous and Future Networks (ICUFN 2017). Currently, he serves as an associate editor for the IEEE Transactions on Communications, an editor for the IEEE Transactions on Green Communications and Networking, an editor for the IEEE Wireless Communications Letters, and an associate editor for IEEE Transactions on Machine Learning in Communications and Networking. He was the TPC co-chair of the Wireless Communications Symposium of IEEE Globecom 2021, and actively serves as (senior) program committee members/reviewers for Conference on Neural Information Processing Systems (NeurIPS), International Conference on Machine Learning (ICML), International Conference on Learning Representations (ICLR), International Conference on Artificial Intelligence and Statistics (AISTATS), International Joint Conference on Artificial Intelligence (IJCAI), and AAAI Conference on Artificial Intelligence (AAAI). He is a member of SpectrumX, an NSF Spectrum Innovation Center.
Education
B.S. Tsinghua University, China, 2002
M.S. Tsinghua University, China, 2004
Ph.D. University of California Los Angeles (UCLA), 2009
"I'm fascinated by the power of machine learning, communications, and networking."