Prasanna Balachandran
Assistant Professor
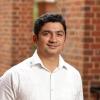
About
My interests are in materials informatics, density functional theory, machine learning, bayesian inference, and optimal design methods applied to accelerate the search and discovery of novel 2D materials, metallic alloys, ferroic and electronic materials.
Research Interests
Density Functional Theory
Materials Informatics
Machine Learning/Active Learning/Adaptive Learning
Magnetic Materials
Ferroic Materials
Electronic Materials
Selected Publications
Accelerated HKUST-1 Thin-Film Property Optimization Using Active Learning
L. HUELSENBECK, S. JUNG, R. HERRERA DEL VALLE, P.V. BALACHANDRAN, AND G. GIRI, ACS APPLIED MATERIALS AND INTERFACES 13, 61827–61837 (2021)
A Bayesian Approach to the Eagar-Tsai Model for Melt Pool Geometry Prediction with Implications in Additive Manufacturing of Metals
B.J. WHALEN, J. MA, AND P.V. BALACHANDRAN, INTEGRATING MATERIALS AND MANUFACTURING INNOVATION 10, 597-609 (2021)
Machine-Learning-Enabled Prediction of Adiabatic Temperature Change in Lead-Free BaTiO3-Based Electrocaloric Ceramics
M. SU, R. GRIMES, S. GARG, D. XUE, AND P.V. BALACHANDRAN, ACS APPLIED MATERIALS AND INTERFACES 13, 53475-53484 (2021)
Density functional theory study of chemical pressure in multicaloric MTX compounds
T.Q. HARTNETT, V. SHARMA, R. BARUA, AND P.V. BALACHANDRAN, APPLIED PHYSICS LETTERS 118, 212408 (2021)
Machine learning for materials design and discovery (Editorial)
R. VASUDEVAN, G. PILANIA, AND P.V. BALACHANDRAN, JOURNAL OF APPLIED PHYSICS, 129, 070401 (2021)
Courses Taught
MSE 4592/6592 (Special Topics: Introduction to Materials Informatics)
SPRING 2020
MAE 3420 (Computational Methods in Mechanical & Aerospace Engineering)
FALL 2019
MSE 4592/6592 (Special Topics: Introduction to Materials Informatics)
SPRING 2019
MSE 2090 (Introduction to Materials Science)
FALL 2018
Awards
Research Excellence Award, University of Virginia
2020
DARPA Young Faculty Award
2020
Rising Star, Computational Materials Science (Top 20)
2019