Xinfeng Gao
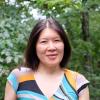
About
Xinfeng Gao's research is focused on the development of high-performance computing (HPC) computational fluid dynamics (CFD) algorithms for a wide range of applications in aerospace and mechanical engineering, involving shock waves, turbulence, combustion, plasma, and multifluids. Her research is categorized into three areas:
- The development of numerical algorithms for complex partial differential equations (PDEs),
- HPC and software engineering,
- The development of enhanced CFD modeling & simulation (M & S) tools by data analytics.
Integrating these research capabilities together, she has been collaborating with national labs, universities, aerospace industry and software companies to transform research across scientific disciplines for solving complex problems of national and societal interest. Her research has been supported by the National Science Foundation, Air Force Office of Scientific Research, Department of Energy Laboratories, e.g., Lawrence Berkeley National Laboratory (LBNL), Lawrence Livermore National Laboratory (LLNL), etc, and Aerospace Industry, e.g., the Boeing Company, the Woodward Inc., etc.
Dr. Gao earned her PhD in Aerospace Science and Engineering from the University of Toronto Institute for Aerospace Studies in November 2008. After graduation, she became a postdoctoral scientist working with two groups in LBNL from January 2009 to August 2011: the Applied Numerical Algorithms Group and the Center for Computational Sciences and Engineering. Prior to joining UVA in late July 2023, she was a professor at Colorado State University in the Department of Mechanical Engineering, where she started as an assistant professor in mid-August 2011 and established the CFD and Propulsion Laboratory. She spent her sabbatical from August 2018 to May 2019 reinvigorating her research by working with the Mathematical Algorithms & Computing Group in LLNL's Center for Applied Scientific Computing.
Education
University of Toronto, Aerospace Science and Engineering, PhD 2008
Lawrence Berkeley National Laboratory, Applied Numerical Algorithms, Postdoc 2009-2011
"A Simulation Is Only As Good As Its Algorithm."
Research Interests
Courses Taught
Awards
Featured Grants & Projects
RIG Chair: Xinfeng Gao, MAE
Committee: Chloe Dedic (MAE); Patrick E. Hopkins (MAE, MSE, Physics); Allan I. Harrison (Chemistry); Elizabeth Opila (MSE); Chris Paolucci (Chem. Eng.)
Faculty interested in joining or learning about our RIG project are welcome to get in touch at hi.rig@virginia.edu