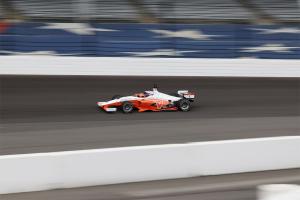
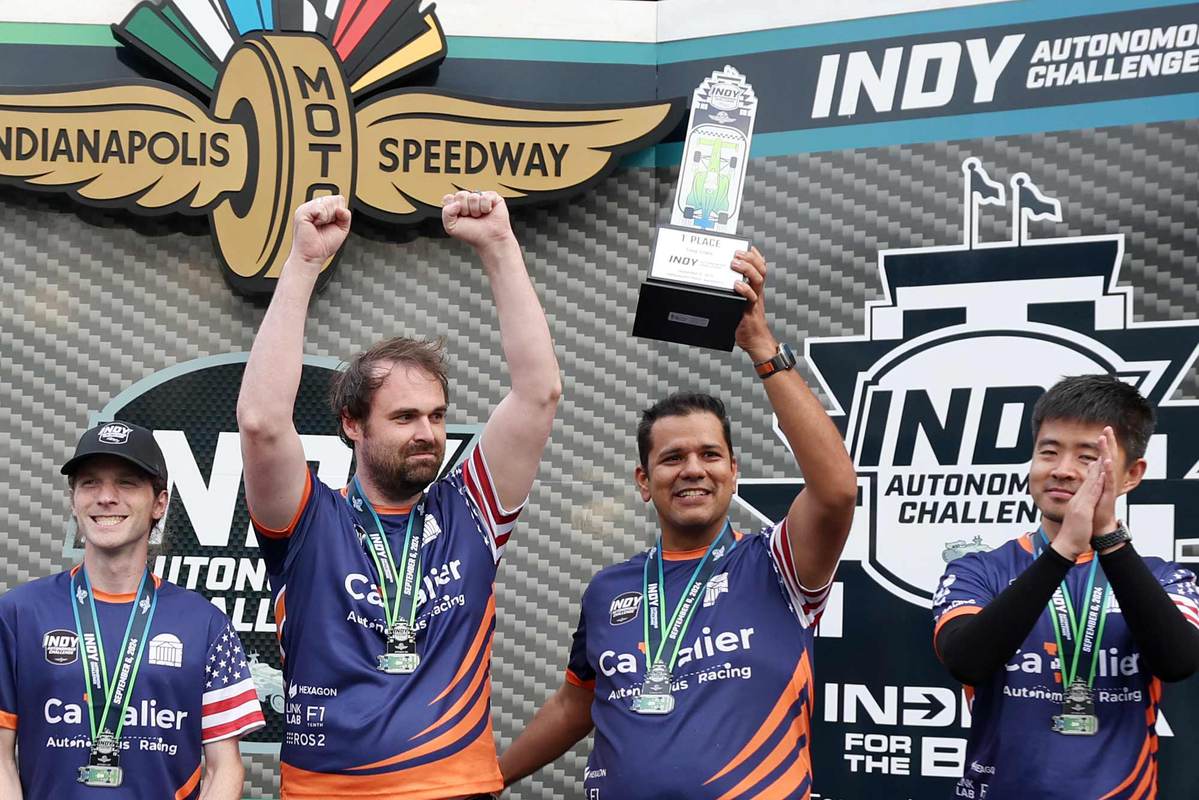
In case you missed it, when it comes to mixing track racing and computer science, the University of Virginia School of Engineering and Applied Science now has the fastest autonomous racecar in the world.
The exciting algorithmic display of speed, control and agility played out on the oval at the famed Indianapolis Motor Speedway on Sept. 6.
The Cavalier Autonomous Racing team — also known as “CAR” or “CAVCAR” for short — did two things that amazed viewers in the latest Indy Autonomous Challenge:
First, CAVCAR followed a beautifully programmed racing line to achieve the 171-mph average lap speed the team clocked to win the speed trial leg of the event. That average speed was a record, as was the team’s top speed of 184 mph before finishing their laps.
UVA's team principal said that a small but insightful tweak gave the team an edge.
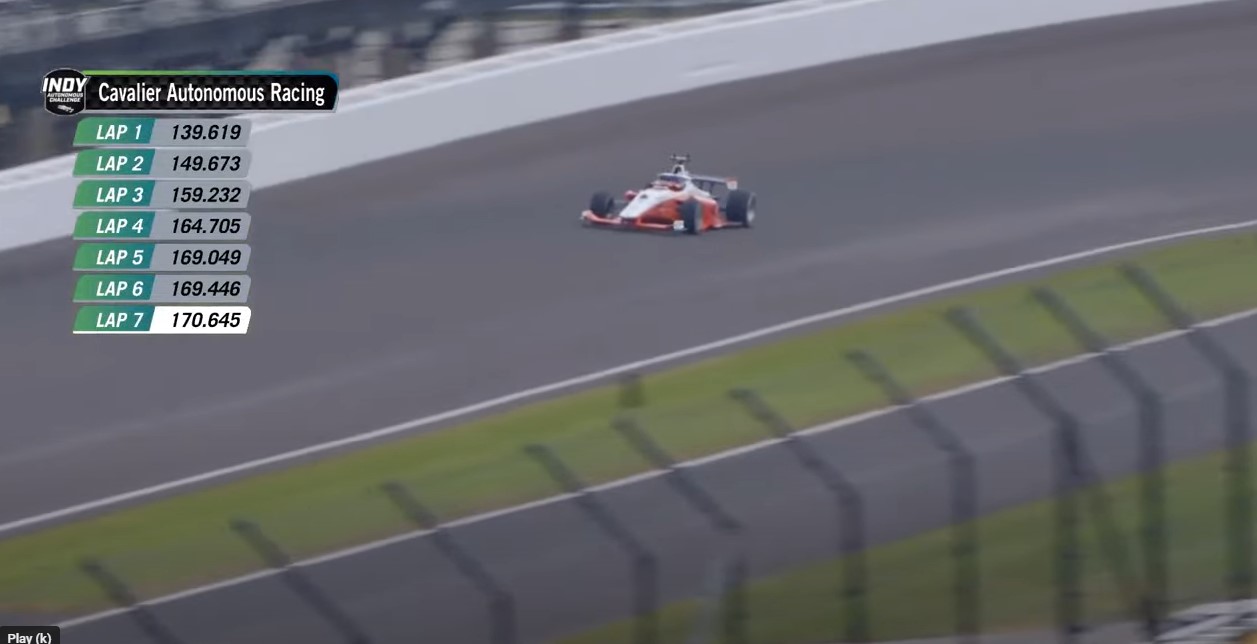
“It's a very simple adjustment to the way we mathematically model the dynamics of the vehicle and account for the suspension geometry and vehicle alignment, but it hasn't been done before,” Madhur Behl, associate professor of computer science and systems information engineering, said. “We haven't seen any literature on this before.”
In addition, the team’s Dallara AV-24 racecar averted what seemed to be a certain crash during the high-speed passing maneuver portion of the contest, breaking on a dime and altering course, then completing the pass successfully.
Incredibly, the team did all of this with only four Ph.D.s on its roster, compared to competitor teams with dozens.
What do these feats mean for the advancement of artificial intelligence and the future safety of vehicles on the roads? Let’s rewind the tape and take a closer look at what the University of Virginia students and their robot so skillfully accomplished.
Speed, Tires, Rain, Control
After a week of glaring sun, a heat which barely fazed the track’s yellow-shirted workers, UVA paraded CAVCAR through Gasoline Alley on race day to its pit position, accompanied by a cool breeze and the U2 song “Beautiful Day.”
And it did turn out to be a beautiful day, even after an afternoon downpour that required the teams tarp their robots and the maintenance crew dry the track.
It was a risk: Should UVA, which hadn’t completed its seven minutes of lap time yet, plan to go as fast as they knew their car was capable, or should they play it more conservatively? Behl said raceway officials were confident about the readiness of the course, so he trusted them.
The other thing that gave Behl and his students confidence was their new vehicle dynamics mathematical model.
“All of racing comes down to how the tires make contact with the tarmac, because that's what m’boy Newton has said will push the car forward.”
Behl explained that the cars, if allowed to roll on their own, would automatically pull left going into a turn because of the way the suspension of the race car has been set up. This suspension alignment is called a “camber adjustment” to an inward or outward tilt of a tire.
“At the oval, the car is set up to have a camber that makes it easy to turn left, since that is what you need to do throughout,” he said. “However, when you want to drive straight and fast, the programming must compensate the steering to counter this left pull and keep the car stable in a straight line. The adjustment we made, was a simple, but clever way to account for the camber in our vehicle dynamics modelling.”
Jingyun Ning, a Ph.D. student in the Department of Electrical and Computer Engineering, and CAR’s vehicle dynamics and control team lead, said the camber and roll adjustment modeling will be the core of an upcoming paper.
“They allowed us to correct for the mismatch between what the model thinks the car should do and what the data is telling us the car is doing,” Ning said. But, he added, “Because of environmental variables such as aerodynamic drag, downforce and the complexity of nonlinear tire-road interactions, we understand the car’s model can never fully capture the real-world physics of its motion."
As can be seen in the livestream recording starting at around 3:28, UVA, the No. 9 car, strategically warmed up — then went for it, executing a final winning lap time of 52.62 seconds. Unlike other teams, Virginia’s strategy was to chart a smooth, continuous course. The more perfect the line, the less the need for steering.
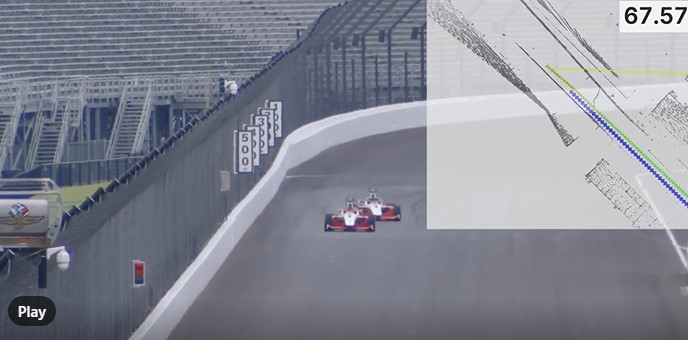
Behl addressed the significance from a vehicle safety development perspective. While wet asphalt wasn’t a risk factor in the race, he said that understanding how to control cars at racing speeds is relevant for passenger autonomous driving because cars traveling at 60 mph in rainy conditions, for the purposes of tire grip and braking distance, might as well be traveling at 100 mph or more.
“All of racing comes down to how the tires make contact with the tarmac,” Behl said, “because that's what m’boy Newton has said will push the car forward.”
Braking, Passing, Agility, Dynamics
The other thing of beauty UVA pulled off on the racetrack was a safety maneuver CAVCAR performed during the passing competition. Starting at about 5:08 on the livestream, UVA had to go around UNIMORE's car, traveling at 80 mph. From the view as they’re coming into the straightway, it seemed as if they were locked bumper to bumper.
CAVCAR’S onboard electronics feature three LiDARs, two radars and six standard cameras, as well as four GNS (GPS) antennae. Together, sensors and software had to decide whether to try to complete the pass.
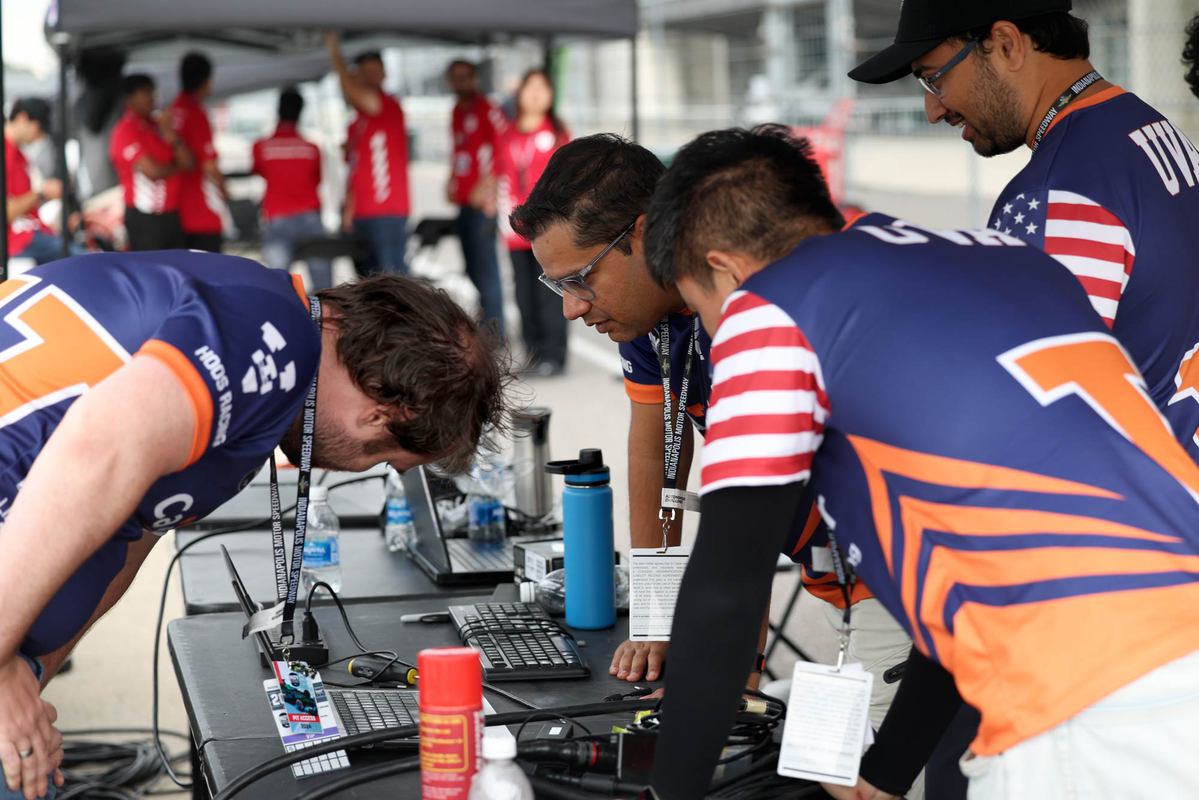
“Coming into the overtaking zone, our perception system locked on to the opponent but had a slight error in predicting their trajectory as they exited turn 4,” Behl said. “No autonomous vehicle is immune to the mis-prediction of another agent’s behavior. So the motion planner evaluated based on the incorrect prediction that an outside pass was still possible if it accelerated then.”
The No. 9 car accelerated to 90 mph, closing the gap for an outside pass.
But the planner quickly re-evaluated as the opponent veered towards the outside, making the pass unsafe. CAVCAR slowed down from 91 mph to 65 mph to avoid a certain collision.
“Then, it identified that an inside overtake was still feasible, with the opponent staying on the outside line, and our car executed the inside pass safely,” Behl said.
In other words, CAVCAR wisely backed off to live for another lap. It also “learned” from the incident.
“During the next lap, in a different part of the track — at the exit of turn 2 — the planner applied what it learned from the turn 4 encounter, anticipating the opponent’s outside line and opting for a safe inside pass,” Behl said.
The breakthrough in making the AI work so well with the newly upgraded AV-24 car hardware, including an updated “drive by wire” actuation system that replaces mechanical linkages with electronic signals, happened during practice runs at Kentucky Motor Speedway.
...We were able to see our passing opponent, but intermittently they would disappear on our screen, or there would some ghost reflections on the track.
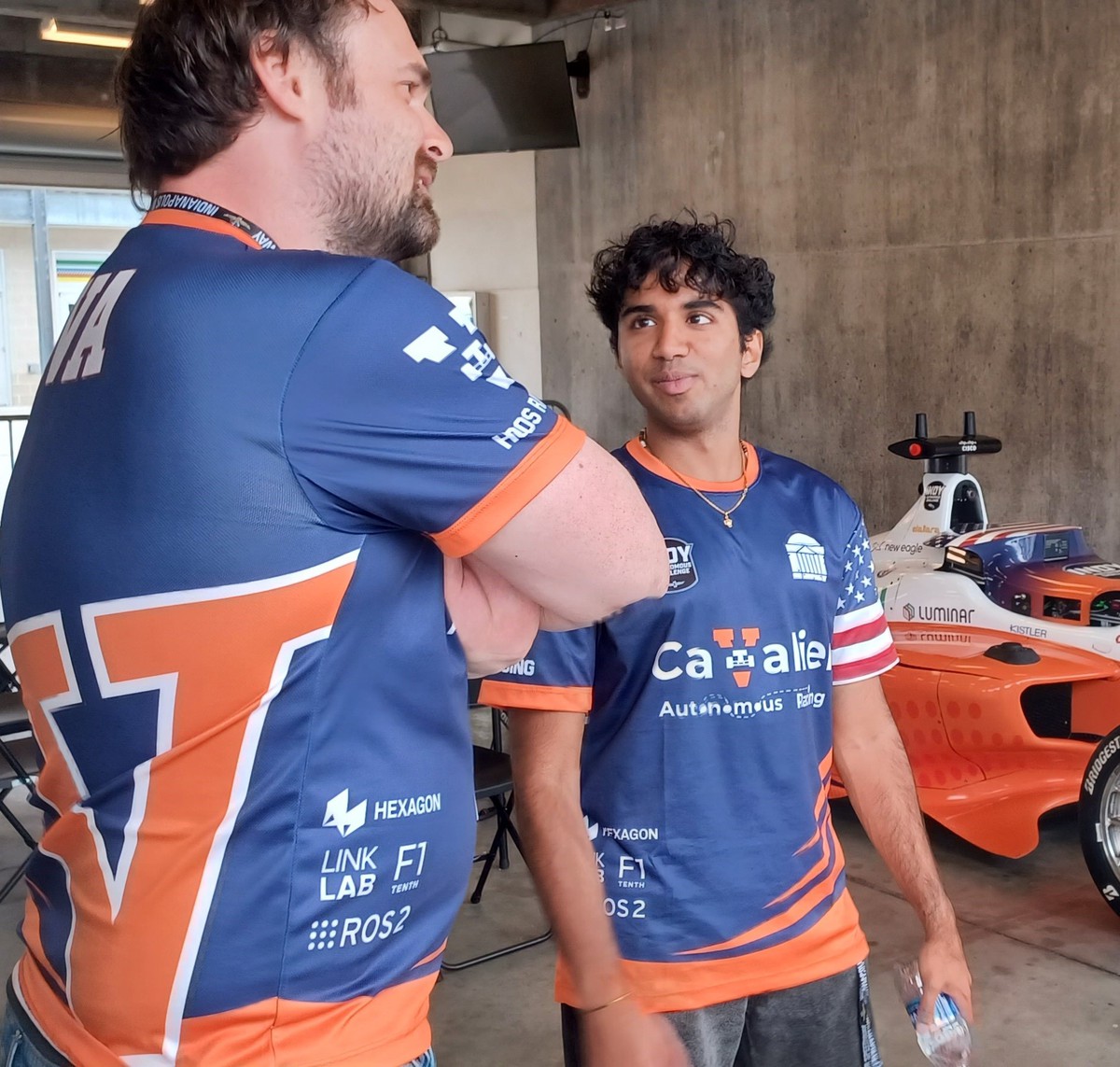
Successful track time is essential for teams hoping to qualify for the Indy Autonomous Challenge.
“With our previous perception AI, we were able to see our passing opponent, but intermittently they would disappear on our screen, or there would some ghost reflections on the track,” said Trent Weiss, a computer science Ph.D. student, former perception team lead and current senior crew member.
Weiss recently submitted a paper for publication on the software upgrade. He anticipates graduating in the spring. He worked closely on the upgrade with Shreepa Parthaje, a computer science undergraduate who has risen to perception team lead.
“We had been sending my students who were in Charlottesville, Trent and his team, the perception team, the data from our Kentucky track, uploading every single night from the Airbnb where we were staying,” Behl said. “They were training essentially a new car detection algorithm for the LiDar on this data. We were getting a little impatient as qualification loomed: ‘We need to qualify now, where is this newly trained model?
“Then Trent shoots a message to the team, and he says, OK, the model looks like it's finished training here. And Amar [Kulkarni], the co-technical team lead, and I literally just pulled this new model from the cloud and tried it out on track. And it's like an instant improvement.
“That's AI.”
The Social Significance: Greater Trust in Tech
Behl praised all his students, including Aron Harder, another computer science Ph.D. student who does double duty as the safety and autonomy team lead, and the co-technical team lead.
“Without Aron, our car cannot run, as simple as that,” Behl said. “And his research [on helping autonomous cars better plan in making left turns at intersections] is tied to safety of self-driving cars. It's not even directly related to racing yet.”
Though many in the racing community are underwhelmed by the idea of AI replacing human drivers, entrepreneur Scott Jasek, a partner in the company Indy Racing Experience, said attitudes are slowly changing.
Jasek, an ex-Budget Rental Car executive, has partnered with racing legend Mario Andretti and other professional drivers to provide customers, sitting inside a two-seater cockpit, the thrill of being driven at 200 mph down the straights of the Indianapolis Motor Speedway.
“In our first discussion about autonomous, [Andretti] was initially freaked out about it,” Jasek said. “We then got into the discussion of humans communicating on the racetrack. In here at the Indianapolis Motor Speedway, at the two ends of the track, you have human spotters. Mario made the comment, ‘I'm afraid of spotters because I don't know if they're paying enough attention to what's going on.’ And I said, ‘That's another form of how AI can help. We could eliminate spotters, and the cars can communicate so they don't run into each other.’ So that's when he started to warm up to the idea.”
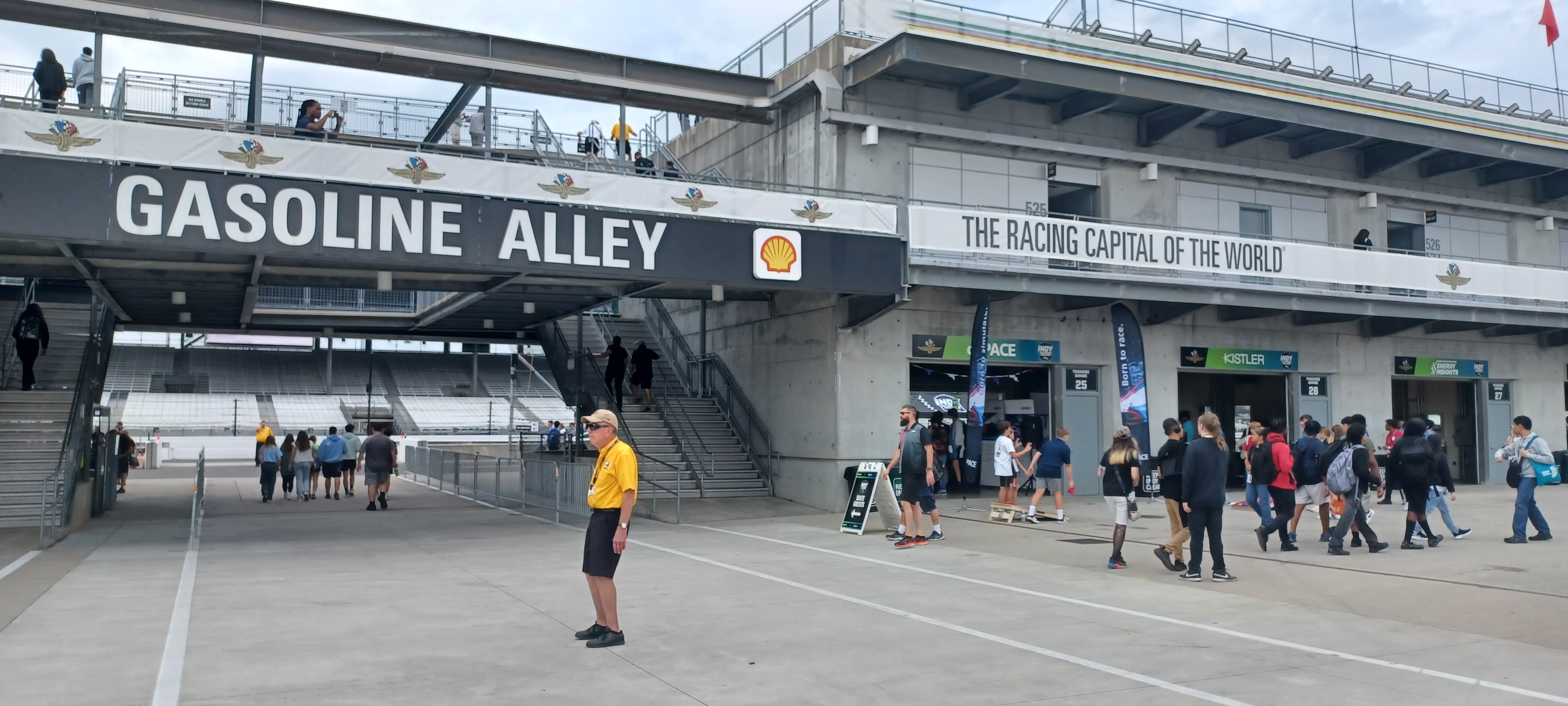
Like Andretti, many of the men and women who worked security on the Speedway track during the Indy Autonomous Challenge were among the voluminous group of Americans who may one day soon greatly benefit from the assistance: senior citizens.
One of the watchful sets of eyes belonged to Ron Luther, a Columbus, Ohio, accountant now in his 70s.
“Well, obviously, the world's going to change,” Luther said. “You have to recognize that. But I've seen so many changes in my life. This is just going to be another one of them.”
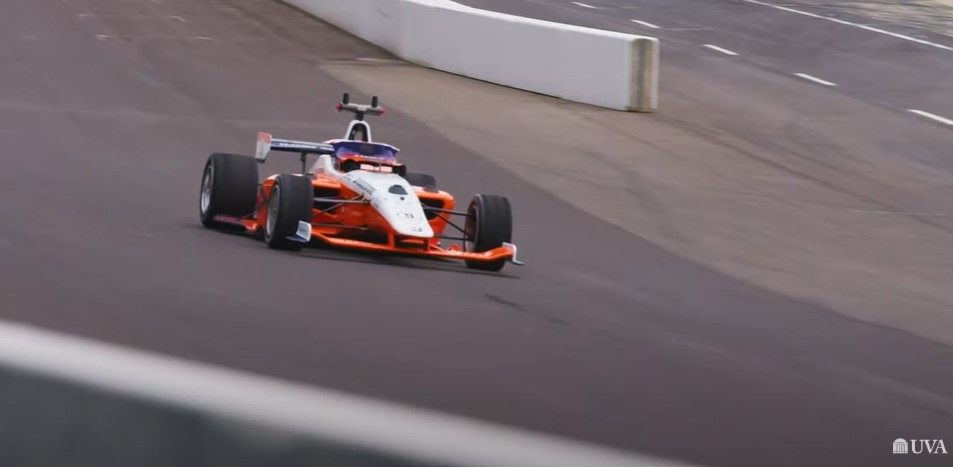