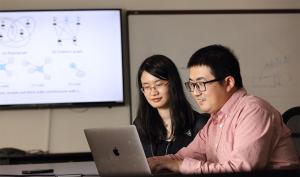
Throughout the COVID-19 pandemic, many consumers relied on credit to see them through. NerdWallet’s annual look at credit card and other forms of household debt reveals that overall debt and loans for housing, cars and education increased between June 2021 and June 2022.
But nearly a quarter of Americans lack the ability to access credit, compounding the financial pain of lost jobs, reduced wages and rising prices. According to financial services firm Morning Consult, around one in four American adults rely on high-fee alternative financial systems such as money orders, payday lenders and check cashing services. Ten percent of U.S. adults do not have checking or savings accounts at all.
For these consumers, getting a loan or a credit card is difficult if not impossible. To address the disparity, lenders are turning to machine learning tools that can bring together a range of data including paychecks, employment history, age, financial behavior and social safety nets. With a more holistic and balanced picture of creditworthiness, lenders can make financial products inclusive of more consumers.
Jundong Li, University of Virginia assistant professor of electrical and computer engineering, computer science and data science, is working with a research team at J.P. Morgan Chase to explain how the firm’s machine learning models reach a decision on an applicant’s credit-worthiness. Li earned small grants from the company’s AI Faculty Research Awards Program in 2021 and 2022 to develop interpretation tools that can help ensure algorithms treat people fairly.
Moody’s affirms that machine learning methods are more accurate than standard statistical approaches because they use a broader set of variables and can find indirect relationships between a consumer’s financial picture and default risk. On the other hand, machine learning models remain a “black box” and the results they generate can be difficult to interpret.
“We want to give Chase’s AI research team a useful tool to trace back and audit the machine learning model’s reasoning process,” Li said. “Financial companies and their stakeholders really want to understand why a model assesses applications as risky or safe.”
The J.P. Morgan Chase grants move Li toward that goal by providing synthetic data derived from real-world scenarios. “It’s a win-win,” Li said. “They can test new algorithms and we can see the impact of our developed solutions.”
Li’s 2021 grant award focuses on the usability, interpretability and fairness of causal effects learning from data in financial applications. To assist in this effort, Li turned to Jing Ma, a Ph.D. student of computer science, who works with Li and Aidong Zhang, William Wulf Faculty Fellow and professor of computer science, biomedical engineering and data science.
Ma’s research focuses on causal inference and graph mining, to which she brings extensive research experience in active learning, crowdsourcing and distributed computing. For the Chase AI grant, Ma is studying the causal effects of different policies that financial companies follow when assessing credit worthiness, including an applicant’s geographic and demographic data.
“We are investigating how a policy affects different users, and what kind of policy yields a better application result for consumers,” Ma said.
Ma’s research will help explain why an application was denied. Ma can make a change in the policy – a treatment, in data science terms – and re-submit the loan application to see if the decision changes. By repeatedly running the loan scenario with different treatments, Ma can help Chase better educate prospective borrowers on how to strengthen their applications.
Ma also uses this technique to ensure algorithms make the same assessment for borrowers with a similar financial profile. Ma can manipulate the treatment scenario to change the applicant’s gender from male to female, or the applicant’s race from Caucasian to Black (while keeping all other variables and conditions the same), to see whether and how the demographic change affects the loan decision.
“The Chase grants offer us a great opportunity to combine human intelligence into a predictive model and help people make good decisions,” Ma said. “I see a lot of potential in this area of research. This is what motivated me to pursue my degree in computer science and why I believe that machine learning is the most important branch in computer science right now.”
Li and his research group members are working on several projects to help people make informed decisions and to promote fairness-aware artificial intelligence. Earlier this year, Li earned a prestigious National Science Foundation CAREER AWARD to better understand cause and effect in human decision-making in the era of big data. His work has the potential for broad applications in education, health and medicine as well as the financial system.
“I want to make machine learning or AI algorithms more accountable and ethical,” Li said. “I think fairness is very important for algorithms that affect people’s daily lives. That’s the part I care about.”
Li is among five faculty in the Charles L. Brown Department of Electrical and Computer Engineering who earned CAREER awards, demonstrating the department’s research strengths in machine learning, cyber-physical systems and hardware for AI and the internet of things.