Continuous Domain Compressed Sensing
The theory of compressed sensing (CS) has revolutionized the area of signal processing by overcoming classical resolution limits. However, current discrete CS formulations are often restrictive and result in model mismatch in imaging applications, where the recovery of a continuous domain image from continuous domain measurements is considered. We introduce a novel structured low-rank matrix completion formulation, where the recovery of a continuous domain image from its sparse measurements is considered. In particular, we reformulate sparse recovery of continuous domain signal as a low-rank matrix completion problem in the spectral domain, thus providing the benefit of sparse recovery with performance guarantees. Fast algorithms that are comparable in complexity to discrete compressed sensing methods are also investigated. This framework improved the state of the art in various applications, including dynamic MRI, parametric imaging, and diffuson MRI. For details, see slides of the tutorial at ISBI 2017 by Mathews Jacob and Prof. Jong Chul Ye.
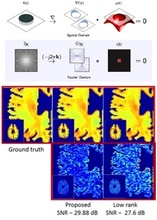