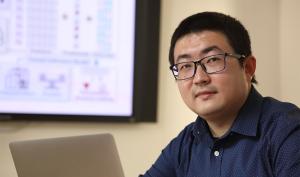
Children attend pre-school and kindergarten to develop the skills they need to thrive. They practice sharing and making friends. They learn to use words and numbers. They gain confidence through movement and self-control. Teachers have an array of techniques to help children develop these skills.
But how should they help students who struggle? There’s no shortage of opinions from educators, parents and policy-makers about effective ways to teach children, based on observations of what happens in classrooms and children’s behavior in and out of school. These observations may be missing something important, however - factors that are unseen, unrecognized or unreported but are powerful influences on a student’s performance.
Jundong Li, University of Virginia assistant professor of electrical and computer engineering, computer science and data science, is conducting research that could help teachers and administrators more accurately determine which learning methods are best for their youngest pupils.
Li has earned a prestigious National Science Foundation CAREER award to better understand cause and effect in human decision-making in the era of big data. Li will use his $600,000 five-year award to develop a suite of sophisticated algorithms and mathematical models, informed by human experience and intuition, to find cause-and-effect relationships in a huge amount of data. His work has the potential for broad applications in public health and medicine in addition to education.
The CAREER program, one of the NSF’s most prestigious awards for early-career faculty, recognizes the recipient’s potential for leadership in research and education. Li’s award recognizes his expertise in data mining, machine learning, and artificial intelligence, which are part of a research strength area for the Charles L. Brown Department of Electrical and Computer Engineering within UVA’s School of Engineering and Applied Science.
“The basic problem here is that machine learning and data mining alone are often insufficient to make decisions for humans,” Li said. “Typically, given a large amount of data, machine learning models can find correlations and then use those correlations to make inferences and predict outcomes.”
Because machines cannot really understand human needs, expectations and behaviors, their predictions and recommendations may be based on spurious correlations.
“We all know that correlation does not necessarily imply causation,” Li said. “In order to make a decision, typically we need to have a better understanding of what is cause and what is outcome. We want to find causal relations between variables at play.” This means creating what Li calls a causal inference model, which quantifies the strength of cause-and-effect relationships between different variables and uses the strongest to make a decision.
Nowadays, research to make machine learning algorithms and models better at reasoning is largely data-driven, Li said. “For my CAREER award project, I want to incorporate prior human knowledge into these algorithms, to give the model the benefit of human wisdom as it processes data and interprets decision-making scenarios.”
Li has had preliminary success in his proposed approach, working in the public health arena supported by a RAPID grant from the UVA’s Global Infectious Diseases Institute. Li collaborated with Daniel Mietchen, formerly with UVA’s School of Data Science and now a researcher at the Fraunhofer Institute for Biomedical Engineering in Germany, to assess the impact of COVID-19 related policies on outbreaks. Three members of Li’s research group assisted with the study.
The team’s model shows how COVID-19 policies such as social distancing affected outbreaks at the county level, taking into account people’s vigilance over the virus over time.
A county government may issue policies to enforce social distancing at an early stage of the pandemic, but if residents in the county tend to be more alert to COVID-19, they likely would have a lower probability of infection. In this case, vigilance is a confounding variable, influencing both the “treatment,” or the policy of social distancing, and the outcome, or the number of individuals who get sick.
Publicly available information online provided an important resource. For example, the team used the popularity of Google searches about COVID-19 at different time periods as a measure of residents’ vigilance. Using this indicator and others, the team developed a framework that captures information from different time periods and handles information among counties to estimate how various policies affected COVID-19 outbreaks. The framework shows the cause and effect of policies at different degrees of specificity, from a category of policies with a certain goal, to a single policy.
The team members presented the results of their study in a research paper, Assessing the Causal Impact of COVID-19 Related Policies on Outbreak Dynamics: A Case Study in the US, published in the proceedings of the Association for Computing Machinery’s Web Conference 2022 in April.
“Our web conference paper captures outbreak dynamics more accurately than statistical methods alone,” Li said. “Additionally, our assessment of policies is more consistent with existing epidemiological studies of COVID-19. This suggests that public health officials can use our framework when randomized controlled trials, the gold standard of cause-and-effect estimation, are not feasible.”
Li’s next step involves collaboration with individuals who are knowledgeable about the application areas, such as medical doctors, public health officials and experts in learning and development. He will also identify publicly available data in the areas of health and education that he can mine to further test and develop his decision-making framework.
Ultimately, Li envisions developing sophisticated algorithms that will pinpoint cause and effect, so physicians can use them to customize treatments based on patient information, and decision-makers can plug the algorithms into their own data systems to deliver policies that improve their constituents’ health, economic well-being and quality of life.