Research
Research
The Naegle lab seeks to understand the regulation and function of tyrosine phosphorylation in complex networks.
Tyrosine phosphorylation is a protein modification that can occur during or after translation of a protein. The phosphate addition to a tyrosine residue, regulated by tyrosine kinases and phosphatases, can result in changes in protein function, regulation and localization. It is key to important cell signaling processes, which are the processes that convert extracellular cues, like growth factors and insulin, into biochemical networks that result in a change to the cell. Tyrosine phosphorylation is specifically utilized in the early events of receptor tyrosine kinase (RTK) networks, which are fundamental to many processes in the development and homeostasis of complex organisms. Improvements in measurement technologies have enabled the ability to detect and monitor tyrosine phosphorylation and now we know that tyrosine phosphorylation is extensive — occurring on thousands of tyrosines in the human proteome.
Given the sheer size of the challenge, we use both computational and molecular technologies to predict and test the role of tyrosine phosphorylation on proteins and in cellular networks. Although we incorporate new mathematical and computational methods as needed to tackle the fundamental problems of our research, those techniques always have a foundation in statistical robustness. Hypotheses are tested in molecular and cellular systems, closing the loop between computation and experimentation.
The questions that drive us:
- How do we increase the capabilities of research to gain new understanding of tyrosine phosphorylation rapidly, i.e. in a high-throughput manner that matches the rate of discovery of these modifications?
- How do we develop new capabilities to understand how these networks act in specific contexts? Cell context refers to the differences we see between tissue types and the states of the network components that lead to differential responses of tissues to the same cue. As a philosophy, we approach network dysregulation that occurs in disease as an alteration in cell context.
Our Research Areas
A foundation of our work is the ability to have proteome information at our fingertips. This includes the current knowledge of tyrosine phosphorylation, quantitative measurements measured on those sites, and related protein annotations. In enabling this research for our own lab, we also construct tools that can be used by the broader research community, with a focus on extendibility and reproducibility.
Kristen Naegle developed ensemble approaches to clustering of biological data in her Ph.D. work that demonstrated that one can INFER FUNCTION OF TYROSINE PHOSPHORYLATION from quantitative measurements of the dynamic changes of network phosphorylation in cells in response to growth factor stimulation. During her post-doctoral work, Dr. Naegle went on to show that robustness in clustering was predictive of protein interactions and INFERRED NOVEL INTERACTIONS IN THE EPIDERMAL GROWTH FACTOR RECEPTOR NETWORK.
A major piece of ongoing work in the lab is to develop methods that will allow us to identify what phosphotyrosines will be recognized by a binding domain. Specifically, we hope to push this area of research into arenas that allow us to predict the relative competition between domains for phosphotyrosine sequences and phosphotyrosine sequences for domains. This information will enable us to begin to predict the consequence of context differences between cells in response to the same extracellular cue. We will feel we have succeeded when these predictions can be used to explain complex network phenomena.
A major barrier to the study of protein phosphorylation is the ability to create phosphorylated proteins for in vitro study. The Naegle lab has been developing a cheap and fast method for producing phosphorylated proteins that capitalizes on observations made of enzymatic specificity.
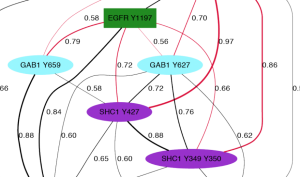
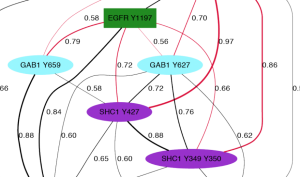
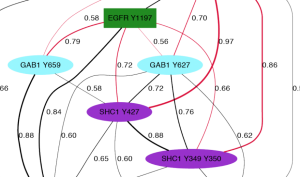