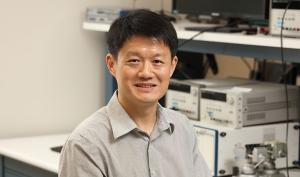
Redial. Speed dial. Mute. Hold. There was a time when that’s all mobile phones could do.
Thankfully, communications technologies mature and transform about every 10 years. Each generation has a feature that proves disruptive in a good way – what engineers call a “killer application” that makes the new technology so attractive it dominates and eventually replaces the old.
In second-generation, or 2G, communications technology, introduced in the 1990s, roaming and clear voice calls across long distances were the killer apps. 3G communications came along in the early 2000s, offering much larger and faster bandwidth; the ability to exchange large data files and emails became the killer app. 4G appeared in the late 2000s, with huge improvements in speed and global mobility, opening up a new world of interactive multimedia experiences such as videos and gaming.
5G debuted in the late 2010s with even faster speeds and improved security. 5G also gave cellular systems the ability to communicate seamlessly with sensor networks that make up a sizable slice of the internet of things composed of billions of connected electronic devices that make our homes, factories and cities smart.
So what’s next? Researchers just started thinking about 6G, and its killer app remains unknown. Among communications engineers working in academia and industry, there is a strong belief that machine learning and artificial intelligence will drive killer applications, giving phones and other devices more abilities to learn and deliver the information and services consumers need through voice, video and touch commands.
Cong Shen, an assistant professor of electrical and computer engineering at the University of Virginia School of Engineering and Applied Science, has earned a prestigious National Science Foundation CAREER award to design 6G wireless communications systems to support machine-learning and artificial-intelligence technologies.
Shen will use his $500,000 five-year award to help communications engineers boost the performance of machine-learning models that drive the internet of things while also protecting data privacy. The CAREER program, one of the NSF’s most prestigious awards for early-career faculty, recognizes the recipient’s potential for leadership in research and education.
Shen leads the Laboratory for Intelligent Communications and Networking, whose members sit at the intersection of machine learning and future communications systems, a research strength of UVA’s Charles L. Brown Department of Electrical and Computer Engineering. Shen’s extensive experience with the companies Qualcomm Research, SpiderCloud Wireless, Silvus Technologies and Xsense.ai makes him uniquely suited to develop machine-learning models that meet communications requirements, and vice versa. Working in partnership with industry, Shen's machine-learning models can be prototyped, standardized and integrated into real systems.
“My CAREER proposal is about building a foundation, an overall framework, for a dramatically different communications system,” Shen said. “The question is how to build wireless communications systems to enable machine learning and artificial intelligence in 6G.”
Since joining UVA Engineering in 2019, Shen has earned a series of NSF and other grants to develop machine-learning technologies tailored to specific industry needs. Shen’s collaborative research projects address such challenges as network reliability and management and how to make better use of the computation power of smartphones, tablets and electronic devices that are part of the internet of things.
“Before my CAREER award, every grant I earned focused on a particular machine-learning technology and communication theme,” Shen said. “The CAREER award allows me to move a number of these machine-learning technologies forward simultaneously.”
Shen will propose new ideas and methods to tackle two general types of machine-learning technologies, based on how the algorithms are trained and refined, and how the training data is collected, stored and utilized.
Consumers have become familiar with their phones’ ability to recognize images and use voice controls. One popular example among bird watchers is Merlin Bird ID, a free app from the Cornell Lab of Ornithology. When spotting an unknown bird, the user answers a few simple questions, uploads a snapshot, and the app names the bird. The app can also identify a bird based on its song, recorded and uploaded by phone.
The algorithms that make this possible are trained on tens of thousands, if not more, images of different birds and billions of song note patterns. Developers use historical data to train the algorithm offline, perfecting its accuracy before it is released to consumers as an app.
A second approach to training algorithms is closer to how humans actually learn things. A good example is how little ones learn to avoid touching things that could burn their fingers. “We don’t train a baby to be ready at year two,” Shen said. “They learn a little bit, test what they’ve learned against different objects, and eventually recognize and avoid things that are too hot to touch.”
Training algorithms using this in-the-moment, experience-based approach is called online learning. Online learning is difficult because a small glitch in communications can create big problems. Going back to the example of a human reacting to something hot: If you hold your hand over a flame, your nerves are supposed to send a “getting burnt” signal to your brain, and your brain ideally sends a signal back to move your hand. If the nerves-to-brain communication loop is not designed well, your brain won’t feel pain; if the brain-to-muscles communication loop is not designed well, your hand will be slow to move.
Applying this analogy to how machines learn, one side of the communications loop is a reward signal and the other is a control signal. A machine gets smarter when both sides have high-quality communications links, without delay, Shen said.